It seems like everybody these days is talking about the expansion of AI use cases in business spaces, and how everyone not learning to use AI tools is falling behind. But many workplaces still don’t have a clear policy dictating how AI can and should be used, especially if they’re in highly regulated industries like finance and healthcare.
In fact, according to Retool’s latest State of AI report, 27.6% of respondents are using AI secretly at work—and of that group almost 35% said that was because their company’s AI policy isn’t clear.
Clearly there’s a gap between the online hype of “AI is taking over everything” and the reality of companies (and individuals) being unsure whether and how employees should use AI, or how to get started with it. This uncertainty can make it tough to get everyone onboard with bringing AI into your organization.
Luckily, you have this guide, where we’ll provide some tips on how to talk to your boss about AI and how to (hopefully) convince them that these tools can be used responsibly, securely, and can deliver a ton of value. While you know your boss better than we do, and what style, format, and tenor tend to land well, these tips will help you prepare for a productive discussion. Plus, we’ve included an email template you can edit to get your conversation started.
Let’s go.
First things first, you’ve got to understand your boss’s point of view. Do you know if they are resistant or enthusiastic? Informed or checked out? That will help color your approach.
Whether they’re an eng lead or the CEO, consider that if they haven’t spent a ton of time actually using LLMs like ChatGPT or Claude, or even some of the AI tools integrated into other apps, they might have a very different perspective on AI than you do. In those cases, much of what they know and understand about AI might not come from experience or research but from the buzz of media (social, mainstream, or otherwise), or from industry chatter. This might mean they are exposed to hyperbolic and hyped-up inputs, or worried, risk-averse ones—rather than specific, useful ways to work with AI.
Without direct experience with AI tools, keep in mind that it’s easy to get lost in the noise. Notably, your boss might have unrealistically high expectations (at first)—or substantial concerns. The former shouldn’t be too difficult to temper, but the latter is trickier. AI is still very new to most people, and is often overhyped and even scary—especially compared to operations, processes, and SaaS tools that are more of a known-known.
Another thing to keep in mind is whether your organization (beyond your pillar or team) has started adopting AI, and how that might influence your manager’s perspective. Is your company crawling, walking, running, flying, or not doing much of anything? Are conversations around AI exciting or hesitant at higher levels of the company?
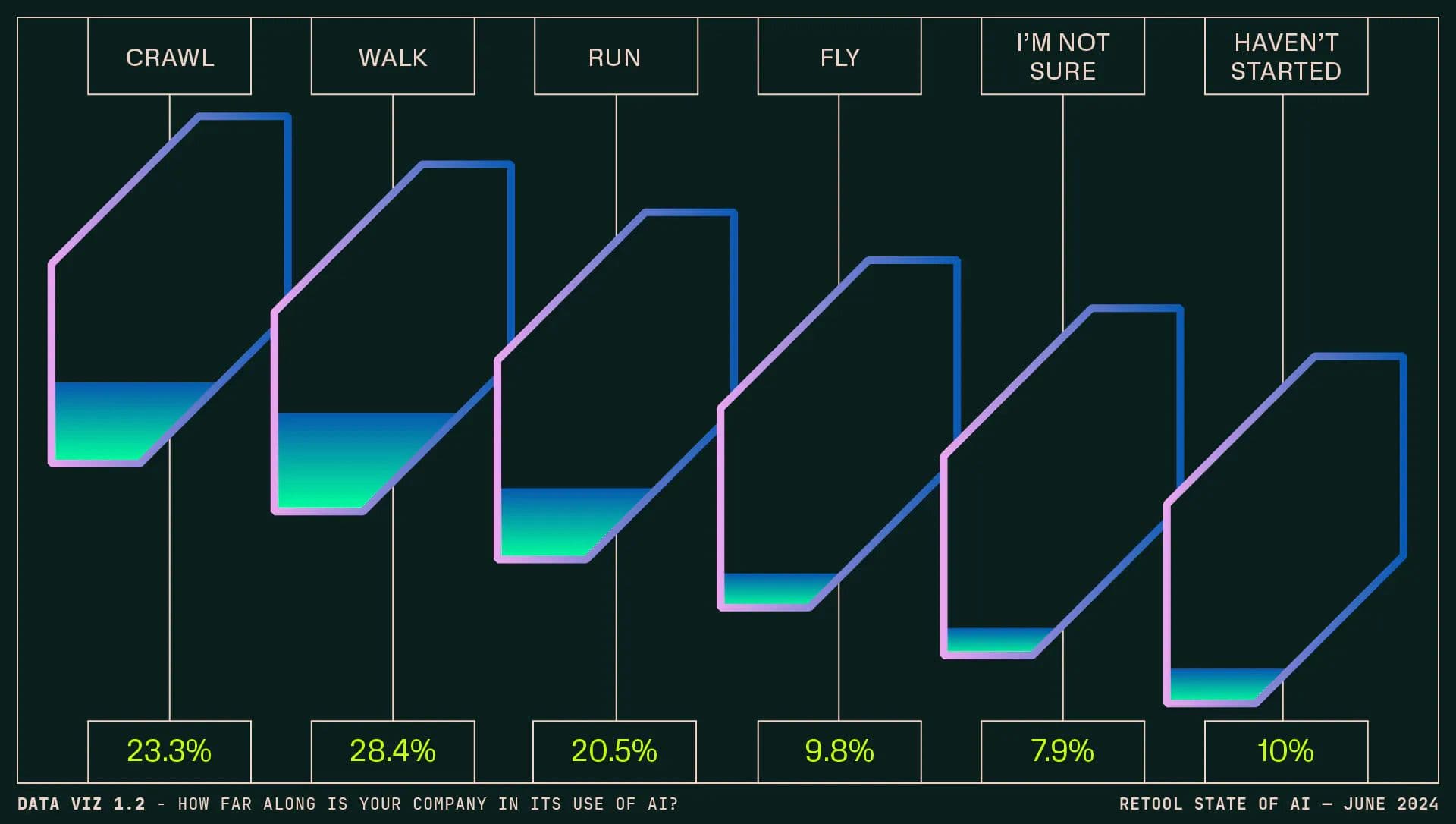
Just over half of respondents in our recent State of AI survey said their company was at the Crawl or Walk stage.
- When you’re in the Crawl stage, you’re just starting to explore AI capabilities and may be using basic tools like ChatGPT for simple tasks. Maybe you’re implementing simple AI-powered chatbots for internal FAQs or using AI for basic content generation (e.g., email drafts, social media posts, etc).
- In the Walk stage, you’re integrating AI into some workflows and seeing initial benefits. This might look like developing AI-assisted customer support tools, using AI for data analysis and report generation, or implementing AI-powered proofreading and editing tools.
- The Run stage involves more advanced AI applications across multiple departments.
- Finally, the Fly stage represents full AI integration throughout the organization, with custom models and innovative use cases.
(And all of these likely still include humans in the loop!)
Since it’s likely your company is still in the Crawl stage or you haven’t even gotten there—don’t worry! All hope is not lost. While that might mean some additional hurdles or a little extra effort on your end to get buy-in, every company has to start (or accelerate from) somewhere.
With empathy toward your manager’s situation and perspective, and clarity on how your company’s current state might influence their desire (or lack thereof) to adopt AI, let’s start to craft a winning AI pitch.
As excited as you may be about the prospect of using AI at work, you should also realize that in many ways, it still is an untested technology. When you’re making your pitch, especially before you have any AI wins under your belt, you need to make sure your view of AI and what you’re promising is realistic and balanced. This will not only be a more truthful pitch, but you might just find that it encourages your audience (aka your manager) to be more receptive and realistic as well.
First off, focus on specific, achievable goals. In order to get buy-in, you’re going to need a specific, necessary, low-risk, and high-reward use case. Whether it’s automating a particular email sequence, summarizing articles and automatically turning them into tweets and LinkedIn posts, or some other business process, it’s much easier to get a “yes” if you can identify exactly how AI is going to help the team.
Hopefully, if you’ve been experimenting with AI, you have some knowledge of the jagged frontier and what sorts of things fall within the bounds of a large language model’s capabilities. At the moment, LLMs are generally most valuable as point solutions that address a specific problem, usually in combination with an internal data set. Think: support bots augmented with a product’s documentation or automated sales outreach seeded with actual past sales communications. However, if you try to jump straight into the deep end and fine-tune your own model for your very first project, you’re probably going to have a bad time.
When you make your pitch, make sure to focus on achievable goals that align with your company’s AI maturity. If you’re working on convincing your boss, this likely means you’re just getting into the “Crawl” or “Walk” stages—so start small and gradually expand AI usage as your collective confidence grows. You’ll likely want to start with an internal use case, as AI apps exposed to the public can carry considerably more risk. (If you don’t believe me, just ask Air Canada, a Chevy dealership in California, or Google.)
If you’re not far enough along to have a specific, high-value use case where you want to apply AI to yet, you’ll need to treat your ask as more of an R&D request, likely time-boxed or restricted in some other way. By setting a boundary (time, money, scope…) on your early AI experiments, you reduce the risk to your manager (that is, it’s clear what saying “yes” amounts to), and you give yourself healthy constraints as well—which will help you create something actually useful.
Despite all your careful planning, your manager may still have reservations—or even hard objections. Here’s some context that can help you address some of the concerns we’ve seen about AI.
At their core, large language models are trained on data taken from all kinds of sources. And because new versions of the models are constantly ingesting more data (sometimes in a major way), there’s always a concern that they’re getting access to data they shouldn’t have and could leak that data to users.
These concerns aren’t purely hypothetical. GitHub’s Copilot and Amazon’s CodeWhisperer have at times been shown to leak valid secrets and other confidential information when prompted in a certain way.This is obviously not great, for many reasons.
The good news is that major AI providers have gotten clearer about what sort of data is used for training and how to opt out. However, there are a few more concrete ways you can mitigate this risk. For example
- Use a service like Amazon Bedrock: Bedrock, a fully managed generative service from AWS, allows you to experiment with AI while making sure that your prompts and responses are processed inside your AWS account so sensitive information never leaves your controlled environment. This is one of ways highly regulated industries are taking advantage of generative AI.
- Self-host an open-source LLM: In addition to the proprietary language models produced by companies like OpenAI and Anthropic, more and more freely available LLMs (Meta’s Llama 3 and others) are also being created. These LLMs can be self-hosted in a variety of ways so that your prompts and responses aren’t shared with the model’s creators and are not used to train future models. And all of them can even be run without an internet connection!
- Check the terms of service: If you don’t want to use Bedrock or self-host an open-source model, confirming the terms of service of some of the model providers might be good enough for what you need. OpenAI and Anthropic both provide information on how they train their models on user data as well as (if applicable) ways to opt out of that training.
Due to how large language models are trained, they’re not usually recalling specific pieces of their training data, they’re doing their best to predict the next word in whatever sequence they’re generating. This means they can generate factually incorrect information, and present it in a very confident way. ChatGPT even includes a warning about this right below the chat input.

Luckily there are a few ways you can combat this hallucination problem and improve the response of your AI models:
- Retrieval-augmented generation (RAG): Instead of relying on the LLM’s existing knowledge base to generate a response for you, especially if you want it to use non-public data in its responses, you could use RAG. Essentially, you can provide additional context (data, documentation, examples, etc.) to the LLM so that it can return a more accurate, specific response. (For more, check out our article on what you need to know about RAG.)
- Keep a human in the loop: Just like you’d review code, tweets, or a blog post produced by a human, you can do the same with what an LLM generates. (Make sure to double check places where the model cites facts or makes assertions!)
- Add a second LLM in the loop: Two heads are better than one, even if they’re artificial. Chaining LLMs together (we recommend using a workflow tool like Retool Workflows to do so) and having the second model read the output of the first and decide whether it’s a good and accurate response to the prompt or whether the response needs to be rewritten can be a great way to address the hallucination issue. The second model has the additional context of a prompt and response and can often infer things about the original prompt that weren’t present, resulting in a better response overall.
There are a whole host of AI vendors (and even different models provided by those vendors) and pricing structures run the gamut. On top of that, many LLMs are priced based on the concept of “tokens”—essentially the units of text that language models process—that can be whole words, parts of words, or even individual characters. Because you never quite know how long a token is, it’s very hard to estimate how much a particular prompt and response will end up costing. But there are ways to prevent your AI budget from running out of control:
- Set (and enforce) a budget: Some AI providers let you set budget limits on specific API keys, allowing you to decide not to spend more than a specific amount on a particular project. This isn’t the case with all providers though, so check whether you can do this with the AI platform you’re using/intending to use.
- Amazon Bedrock (once again): Using AI inference that’s bundled into another service that you already pay for (in this case, AWS) means you can potentially use the budget allocated for that platform to fund some of your AI experimentation without having to get an entirely new budget line item approved.
- …wait: As we saw with the recent release of GPT-4o mini, models are getting cheaper. If you spend some time getting AI integrated into your business but your use case or implementation is too expensive, it likely won’t be for long. Jobs and workflows that cost hundreds of dollars to run a couple years ago are now affordable and our guess is that it’s likely to continue.
Mitigating risk and being considerate of how your AI experimentation and early use cases affect the bottom line of your organization will not only help you get buy-in, but will help ensure your app is as efficient as it can be.
This is where the rubber meets the road. Think this through before you kick off the conversation—because once your boss is warming up to the overall idea, you’re probably going to be asked questions like, “So how would we actually do this?”
As emphasized earlier, you may want to propose a first project that’s internal-facing as opposed to something you’re going to expose to the public. You’ll also want to pick something relatively simple—especially if you’ve never productionized an AI tool before, there are a ton of little things you’ll have to get right as you’re building. Giving yourself a bit of breathing room while you work through those challenges and being realistic about what a win looks like for you and the business can help set your trial run up for success.
OK, you’ve gotten your framing, your pitch, and your implementation sketched. You’re ready to have the talk! Here’s an email template you can use and modify for your hypothetical boss, Sarah. (We recommend using your actual boss’s name… And, you know, signing the email from you…)
Subject: Quick chat about AI tools for our team?
Hey Sarah,
Hope your week is going well! I wanted to bounce an idea off you that I've been pretty excited about lately.
I've been playing around with some AI tools in my spare time, and I think they could be a game-changer for us. I've got some ideas for a small trial run that could really boost our efficiency without any big risks.
Here's what I'm thinking:
1. We start small—just an internal project for a few weeks.
2. We’ll stick to just publicly available data for now. There’s a bunch of helpful applications that we can build without exposing any of our business data to AI models.
3. We check in regularly to make sure it's actually helping (and get a couple folks who are actually going to use it to help us test).
What do you think? Could we grab a coffee chat sometime this week to talk about it? I'd love to hear your thoughts.
Thanks,
Keanan
P.S. I've done my homework on this, and I'm ready for any tough questions you might have!
If you’ve gone through the conversations, gotten the buy-in, and started to build, you’re not quite done yet—you’ve got to maintain the momentum and enthusiasm that could be brewing. Getting a couple interested team members to help you test the app as you’re building is a great way to make sure it will work for more than just you. And it can help get momentum around your AI use case, while giving your manager more folks to gather feedback from if they’re looking for inputs around whether it’s worth it to continue to invest.
Eventually, scheduling frequent demos to larger groups—if your organization is willing to support that—can be another great way to keep up the momentum and get people excited about the possibilities of AI.
The world of AI is constantly developing, and once you’ve gotten buy-in on bringing AI tools to work, you’ll need a platform to help you actually build the apps you need to build.
That’s where Retool comes in.
Retool is an application development platform that can help you build pretty much any sort of business app you can imagine. When you’re ready to integrate AI, Retool plugs into AI resources like OpenAI, Anthropic, Google, Cohere, and Amazon Bedrock to let you test out and query LLMs right inside your app. If you decide you need to use RAG, Retool comes with a built-in vector database where you can put your data and use it as additional context in your AI queries right away.
When you’re ready, Retool AI is there to help you crawl, walk, run and eventually fly with AI. Good luck talking to your boss—we can’t wait to see what you build.
Reader